UAlbany Biostatistician Creates New Method for Investigating Data Collected Across Time
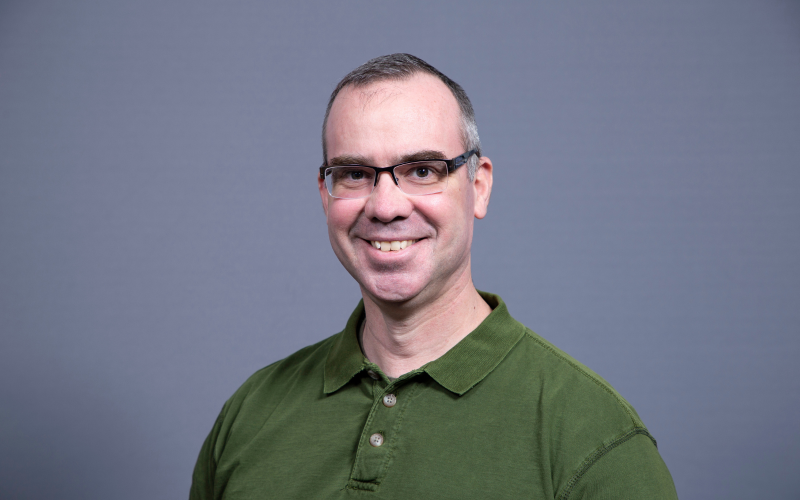
ALBANY, N.Y. (Oct. 9, 2024) — A new statistical method called the Variable Bandpass Periodic Block Bootstrap (VBPBB) was recently developed by UAlbany’s Edward Valachovic, assistant professor in the Department of Epidemiology and Biostatistics at the College of Integrated Health Sciences. The VBPBB helps to create more precise estimates when looking at regularly occurring changes within data collected across time – commonly called time series data.
“The VBPBB is a novel resampling method to investigate the existence and characteristics of periodic variation in time series data that is more efficient, robust and powerful than many existing methods. It has practical applications ranging across diverse fields including public health, environmental science, telecommunications and economics,” says Valachovic.
Data measured across time often results from different influential factors, including those that are periodic. For example, the outside air temperature is not only influenced by the time of day, but the time of year and other factors like cloud cover and rain. Researchers resample the data to learn about the periodic factors, a method known as a periodic bootstrap method. However, this method is hindered by all the factors interfering with each other. The VBPBB method filters time series data based on the period, or corresponding frequency, of the factor of interest– such as daily or yearly variation. Then, researchers can resample from within the filtered data, preserving the periodic characteristics while eliminating interference from other factors, making the estimates based on the selected time periods more reliable.
Now, the VBPBB is being applied to various datasets, including by PhD student Megan Di Maio at UAlbany, who used the method to look at variations in nitrogen dioxide in Los Angeles. Nitrogen dioxide, although it can be naturally occurring, is primarily a man-made pollutant produced by power plants and vehicles. Research shows that it has harmful effects for human health, including links to dementia, breast cancer, decreased cognitive function and increased susceptibility to COVID-19.
“Classical statistics assumes that each sample in a dataset is independent. However, time series data– like temperature changes throughout the year– are related to each other, and traditional methods can miss important patterns,” Di Maio explains. “That’s where periodic bootstrap methods like the VBPBB can assist. This method can also be applied to other data that changes over time, like flu rates or global temperatures, helping us better understand and tackle these issues.”
Di Maio’s study used air quality data taken from a particular spot in Los Angeles, gathered by the Environmental Protection Agency from 2010 to 2022. The information included hourly measurements for nitrogen dioxide levels from the location.
“Los Angeles was chosen because this site had the most complete data compared to other locations in the larger data system, which would allow us to really examine the application of the VBPBB and compare it to other analysis methods,” Di Maio says.
The study looked at the VPBPP in comparison to other periodic bootstrap methods, and found a significant pattern within annual nitrogen dioxide data that the other methods could not identify. In addition, the accuracy levels for the VPBPP results were higher.
“This new statistical method is currently being used in additional research at UAlbany to identify trends and patterns in particulate air pollution, hospitalization usage, energy consumption, and the seasonality of infectious disease transmission,” Valachovic explains. “Megan DiMaio’s work and these other research projects demonstrate the widespread applicability and potential impact of the VBPBB.”
The full report can be viewed in PLOS ONE. The work from this study led to further questions and Di Maio is currently developing an algorithm to help identify periodic patterns of interest within datasets.
“Researchers often check for patterns they are expecting, such as annual or daily variation, but this method with allow them to find patterns in the data that they might not have anticipated,” di Maio says.